Aug 28, 2024
6 min read
From Co-Pilots to AI Agents: Exploring the 5 Levels of Autonomy
The rise of autonomous business processes is transforming how companies operate, driven by AI and automation that enhance efficiency and reduce errors. A McKinsey study shows that AI-driven automation can boost productivity by 30% in just one year.
Autonomy in AI allows systems to perform tasks independently, learning and adapting without constant human input. This mirrors the progression in autonomous driving, where processes have evolved from basic driver assistance to advanced auto(co)-pilots requiring little to no oversight.
A notable example is an insurance company that adopted AI-driven autonomous systems to manage claims processing. By automating this critical function, the company reduced processing time by 90% and increased accuracy in assessing claims. This shift not only streamlined operations, but also improved customer satisfaction by accelerating claim approvals and ensuring more consistent decision-making.
In this blog post, we’ll explore the evolution and levels of autonomy in business automation. We’ll also compare this approach with traditional automation methods and examine how Beam AI is leveraging these advancements.
Let’s start!
The Evolution of Business Automation
Business automation has evolved significantly with the advancement of digital technologies. Initially, automation tools were designed to replace manual labor with machines capable of performing repetitive tasks. As technology progressed, the focus shifted to using computers and software to manage administrative tasks and basic workflows more efficiently.
Early Automation and the Rise of Intelligent Automation
In the early stages of digital automation, tools were developed to assist human workers rather than replace them. These initial automation tools, such as basic scripts and macros, automated repetitive tasks but still required substantial human oversight. For instance, early spreadsheet programs automated calculations but needed manual data entry and monitoring.
As technology advanced, automation tools became more sophisticated, leading to the development of Robotic Process Automation (RPA). RPA could handle complex, rule-based tasks across various applications, reducing the need for human input. Despite its efficiency, RPA had limitations in adapting to new situations or learning from outcomes, which restricted its level of autonomy.
What’s Next? Agentic Process Automation
Agentic process automation is the next step in the automation journey, characterized by systems that can act independently and make choices. These systems leverage AI to understand and interpret data, learn from outcomes, and autonomously adjust their actions to achieve optimal results.
Agentic process automation is characterized by several key features that set it apart from lower levels. Want to understand the key characteristics of APA comprehensively? Read here!
5 Levels of Autonomy in AI
As AI continues to evolve, it’s crucial to understand the different levels of autonomy that define how these systems operate. Each level represents a step toward greater independence and capability, from simple task automation to fully autonomous decision-making. Here’s a detailed look at each level, complete with real-world use cases.
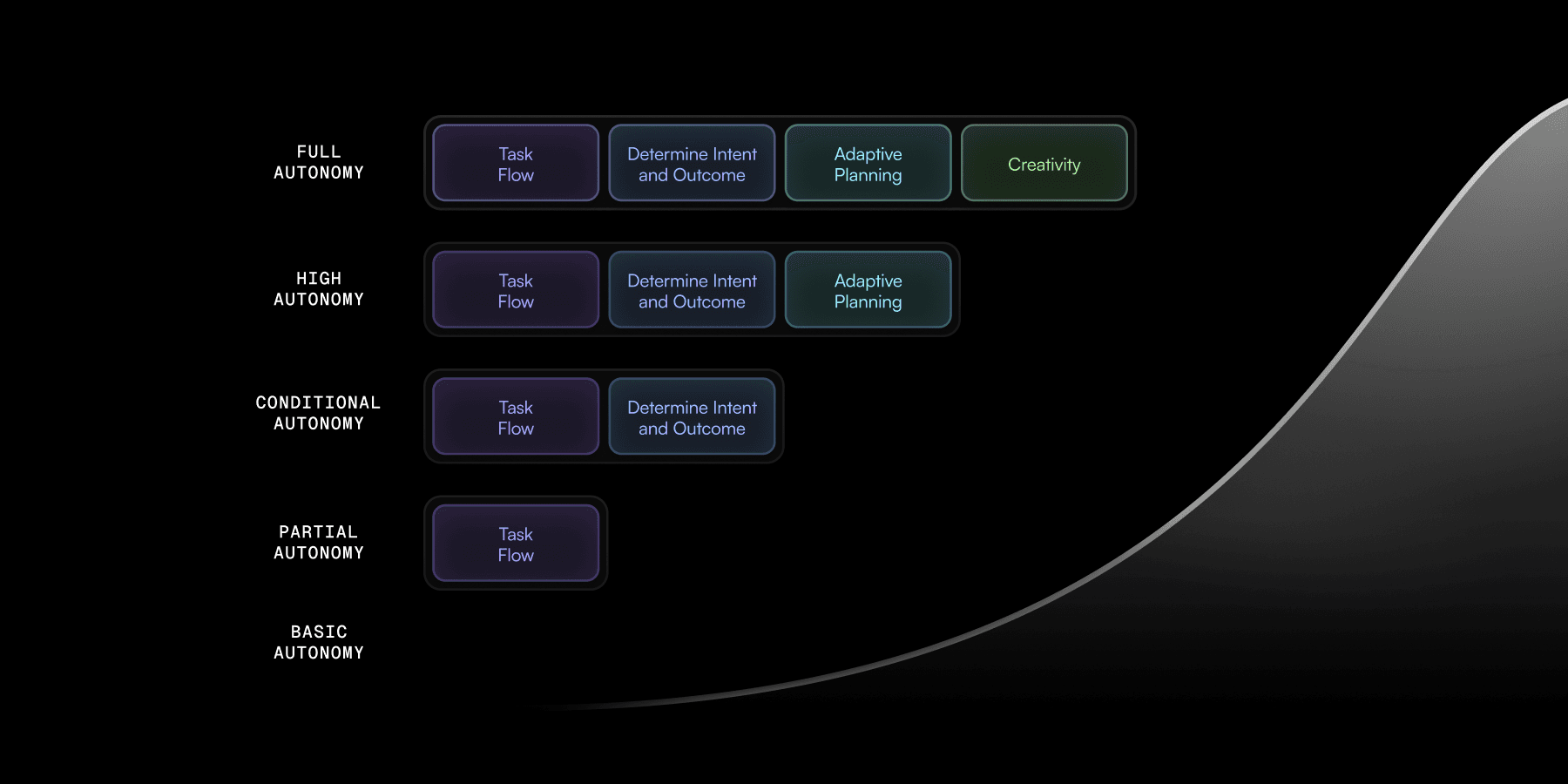
Level 1: Basic Automation
At the most fundamental level, AI systems perform predefined tasks under strict human supervision. These systems follow a set of instructions and execute tasks without any form of decision-making. They’re designed to handle repetitive, rule-based activities where human intervention is needed to initiate and monitor the process.
Use Case: A simple script that automatically formats and organizes data in a spreadsheet whenever it’s updated. The script executes exactly as programmed, with no room for deviation or decision-making.
Level 2: Partial Autonomy
At this level, AI systems can manage certain tasks independently, but they still require human input for more complex or non-standard situations. These systems are capable of handling routine tasks but will defer to humans when they encounter scenarios that fall outside their programmed capabilities.
Use Case: Robotic Process Automation (RPA) software that automates the processing of invoices. While it can process standard invoices on its own, it will flag unusual charges or discrepancies for human review before proceeding, ensuring accuracy and compliance.
Level 3: Conditional Autonomy
Systems at this level can make decisions and perform tasks autonomously, but only under specific conditions. They rely on predefined rules and some level of AI to navigate tasks but require human intervention when faced with situations beyond their programming or encountering uncertainty.
Use Case: An AI-powered diagnostic tool that can analyze medical images to identify common conditions such as fractures or tumors. However, it alerts radiologists to review the results and make final decisions when encountering atypical cases or when additional context is needed for accurate diagnosis.
Level 4: High Autonomy
High autonomy is characterized by systems that can handle the majority of tasks with minimal human oversight. These AI-driven systems are capable of understanding context, learning from data, and making real-time decisions. While they still may involve humans for critical decisions, their need for intervention is greatly reduced.
Use Case: An AI-powered customer service platform that can manage most customer inquiries, including resolving issues and answering questions. Only the most complex or sensitive cases are escalated to human agents, significantly reducing response times and improving customer satisfaction.
Level 5: Full Autonomy
At the highest level of autonomy, AI systems operate entirely independently, making decisions and adapting to new situations without any human input. These systems are self-sufficient, continually optimizing their actions to achieve the best outcomes, much like a fully autonomous vehicle that navigates and responds to its environment without any driver input.
Use Case: An AI-driven document management system that autonomously processes and categorizes incoming documents, extracts relevant data, and updates records in a database. The system efficiently handles routine tasks and data entry, but it escalates complex cases or documents requiring special handling to human operators for further review and decision-making.
To learn more about how AI agents are transforming the job market, check out our article: AI Agents Revolutionizing the Job Market: What You Need to Know
The Beam AI Approach
At Beam AI, we’re merging the best of both worlds: the adaptability and reasoning of AI agents and the reliability of current RPA systems. Our goal is to create smart, context-aware AI agents that seamlessly integrate with your existing processes and keep improving over time.
Beam AI’s agents don’t just automate tasks—they become valuable AI-powered team members, enhancing strategic decision-making and driving business growth.
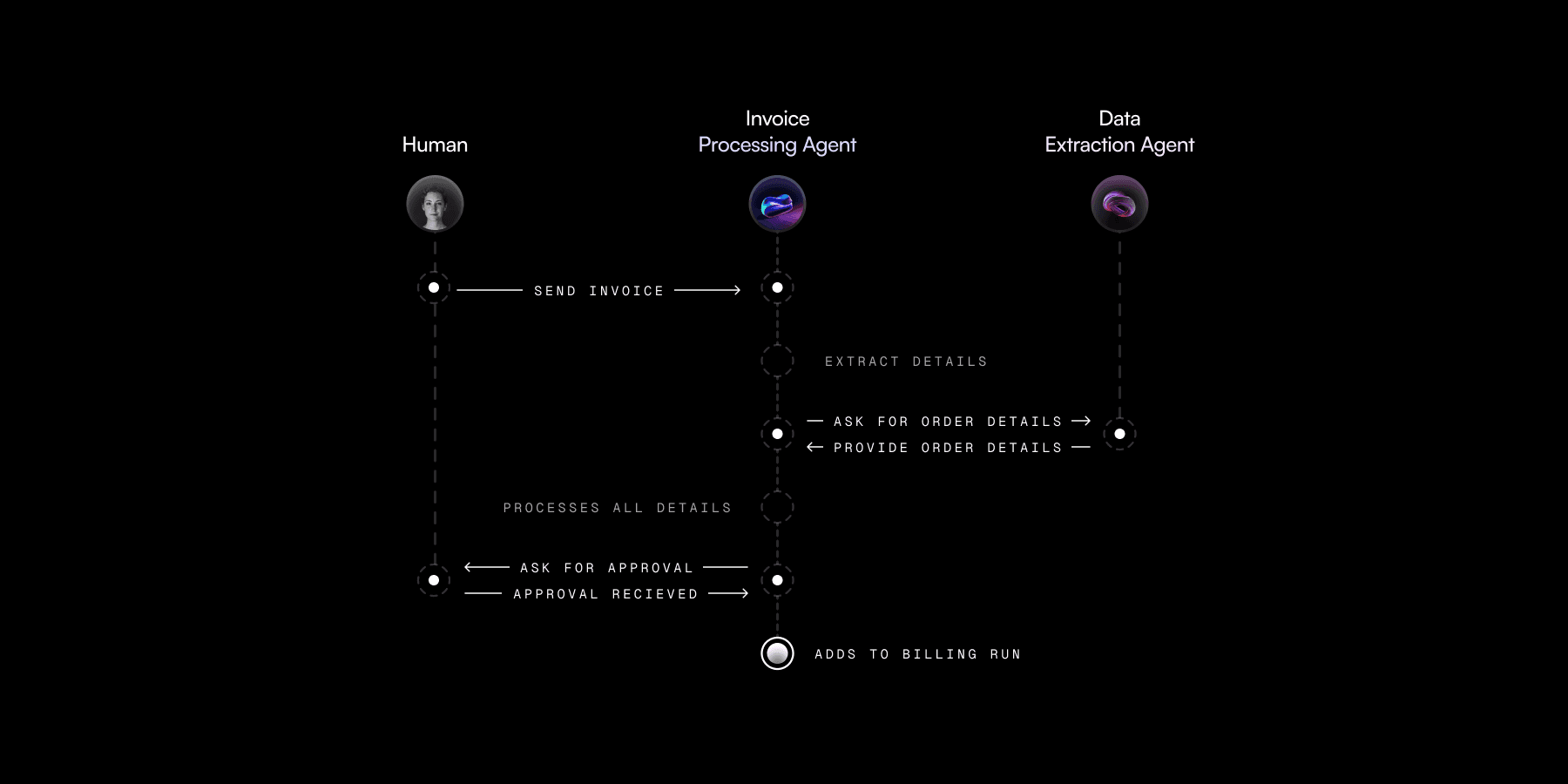
How our AI agents work:
Learning from humans: Our agents grasp your workflows to perform tasks accurately and efficiently.
Choosing workflows: They select the best workflow for each request, based on what they’ve been trained to do.
Ensuring consistency: By following set workflows, our agents maintain reliable results across similar requests.
Seeking clarification: They ask for more information when needed to ensure tasks are done right.
Getting human approval: Critical steps involve human review to guarantee accuracy.
Improving over time: Feedback helps our agents continually enhance their performance.
Join us in revolutionizing business operations with the power of advanced automation. Discover how Beam AI can transform your business today!